Decoding MetaHub’s Anti-fraud Arsenal: Unlocking the Power of BMAS
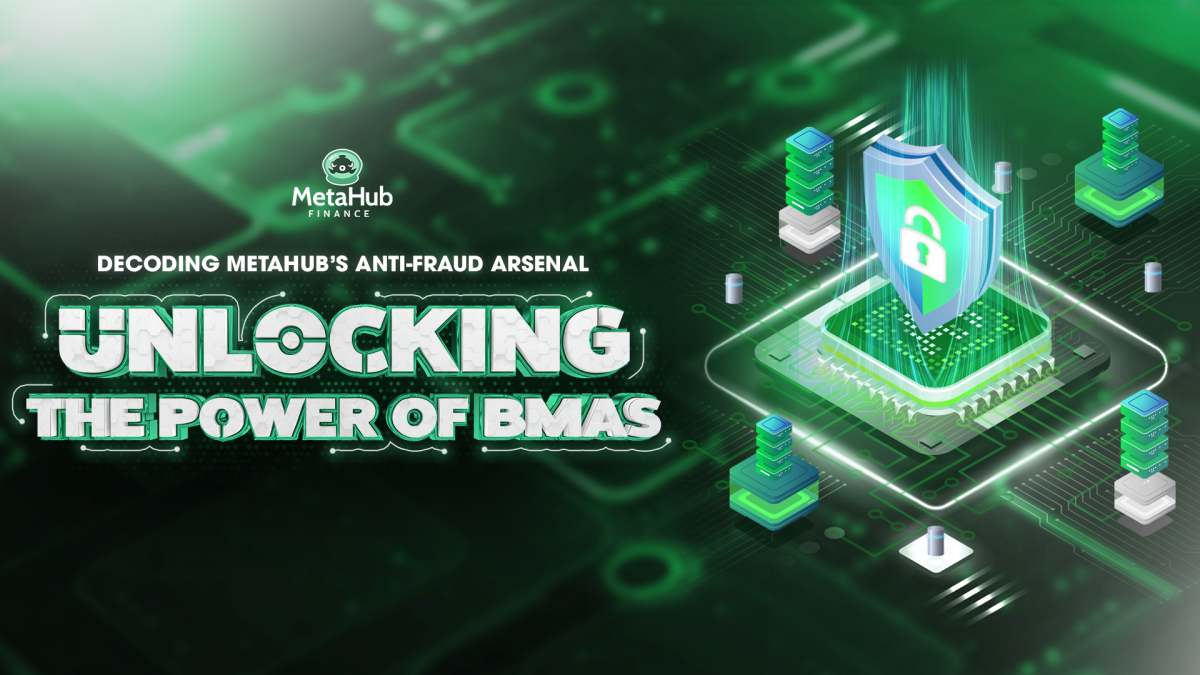
There can be no doubt that security, transparency, and trust are of the utmost importance in the still-evolving world of Web3 and decentralized communities. A fundamental element of these communities is BOT Detection, which is the process of distinguishing between human users and automated bots on websites, applications, or digital platforms. Using two layers of technology, MetaHub has developed a Bot Detection system that incorporates the Bot Mitigation and Anti-fraud System (BMAS). In this article, we will explore BMAS in more detail and see why it has become one of MetaHub’s primary Unique Selling Points (USPs).
What is BMAS and how does it work?
As blockchain technology develops, cyber threats and fraudulent activities become increasingly prevalent. A BMAS is a combination of Bot Mitigation and Anti-Fraud Systems (BMAS) that work together to protect Web3 platforms by reducing the risk of automated bot attacks and safeguarding websites, mobile apps, and visitors from abuse. BMAS system is used to not only stop malicious bots and prevent fraud threats before they impact our ecosystem but also detect fraudulent activity. In particular, to detect fraud, there are several techniques that we are implementing:
- Calculate statistical parameters
- Match data
- Perform Logistic Regression analysis
- Use probability models and distributions
- Mine data to classify, segment, and cluster data to find associations and rules signaling patterns of malicious activities
- Detect fraud with heuristic rules, machine learning, and thresholds
BMAS system will need data to get started. The more data we feed into the system to start with, the more accurate the results will be. Therefore, simulated fraud data is also fed into the system to ensure the volume, quality, and diversity in the analysis process.
The development potential of BMAS
Over the recent years, numerous big enterprises across various industries have opted to utilize BMAS to minimize the risks associated with bot and fraudulent activities. An example is Alibaba Cloud Anti-Bot Service, which offers comprehensive anti-bot protection for web applications, HTML5 websites, mobile apps, and APIs. This solution has already been adopted by notable enterprises like Reddit, Patreon, and AngleList. The BMAS approach holds enormous potential for reshaping behavior management within Web3. BMAS proves its adaptability across various scenarios thanks to its robust machine learning capabilities and real-time monitoring, making it an invaluable asset for enterprises and projects:
- Intrusion and Fraud Prevention
- Enhance User Experience
- Customization and Flexibility
- Support Project Enhancement and Business Development
MetaHub’s BMAS and the reason why it stands out
MetaHub’s BMAS serves as the guardian of the MetaHub ecosystem. It’s a comprehensive system designed to detect and mitigate the activities of automated bots and prevent fraudulent actions within the Web3 platform and it works through five core components:
- User Interaction Monitoring: It monitors user interactions with a system, capturing crucial data like the SoulBound Token and Transaction Data for user authentication and behavior analysis.
- Data Collection: BMAS collects various data points, including IP Address and Location, Citizen Profiling, MetaHub Data, Client Profiling, Fraud Data, and Onchain Transaction data, to form the foundation for fraud detection.
- Fraud Prediction: Advanced techniques, such as tagging, simulations, risk scoring, and actionable suggestions, are used to predict and identify potentially fraudulent activities.
- Fraud Detection: BMAS employs AI/ML models for fraud detection, continually training and testing these models to ensure accuracy. It also fine-tunes its algorithms to adapt to evolving threats and provides real-time insights through a user-friendly dashboard and reporting system.
- Realtime Action: Based on its fraud detection results, BMAS takes real-time actions like allowing safe interactions, blocking high-risk activities, or challenging moderate-risk actions with additional verification.
How BMAS is Applied in the MetaHub DAC Platform
The following information describes our major components in the BMAS system:
1. Feeding the input data to the BMAS System
The data we collect such as IP Address, Browsers, Device Type, Proxy (if used), transaction on-chain, campaign identifier number, types of requests, etc. is used to build “digital avatars” ( act as Soul Bound Tokens ) or fingerprints. For the quest that needs a Sheriff to verify the action, the data is gathered to provide input to the scoring function.
2. Rule generation
Before we have ML/AI, using heuristic rules is one of the most efficient ways. The are two main types of rules that we use in the BMAS system:
- Single-parameter rules, also known as heuristic rules
- Complex rules, including multiple parameters. We can adjust accuracy thresholds to tighten or loosen triggering conditions. The historical data will be used to help us create a confusion matrix based on collected data over the selected time frame and highlight the estimated accuracy rate of those rules.
3. Fraud scoring and analytics
BMAS system leverages machine learning models to assign risk scores to activities or user accounts based on various factors, such as transaction, location, frequency, and past behavior. Higher risk scores indicate a higher likelihood of fraud, enabling us to perform further investigation. Fraudulent actors often collaborate and form networks to carry out their activities. Another machine learning technique we implemented in the BMAS system is semantic graph analysis to help uncover these networks by analyzing relationships between entities (such as users, wallets, transactions on-chain, etc.) and identifying unusual connections or clusters.
4. Risk threshold settings
Finding the optimal risk threshold requires conducting data analysis rooted in the principles of “precision vs recall”. Setting the right limits for Allow/Review/Prevent thresholds depends on this model evaluation metrics. It entails a balancing act involving:
- True positives (the number of fraudsters successfully blocked)
- False positives (the number of legitimate individuals mistakenly blocked)
- False negatives (the number of fraudsters inadvertently allowed) Navigating this intricate balance is crucial for effective risk management in fraud detection and prevention. BMAS system leverages LLM to understand the context and requirements from the natural language of administrators or business partners to suggest the right threshold setting.
In conclusion, Metahub’s BMAS is a revolutionary system that goes beyond conventional behavior analysis. Its flexibility, allowing for tailored prevention levels, gives MetaHub a definitive competitive advantage in the Web3 landscape. By focusing on both security and user experience, BMAS has rightfully taken its place as one of MetaHub’s key techniques, setting it apart as an innovative leader in the field.
———————————-
MetaHub Finance – The Leading Platform for Building the Web3 Affiliate Community
📌 Consult us via: https://linktr.ee/metahubfinance